Home
/ Business & Management / Business Technology / Raising Capital: Credit Tech, Coin Offerings, and Crowdfunding / How Does Platform Lending Work?
This article is from the free online
Raising Capital: Credit Tech, Coin Offerings, and Crowdfunding
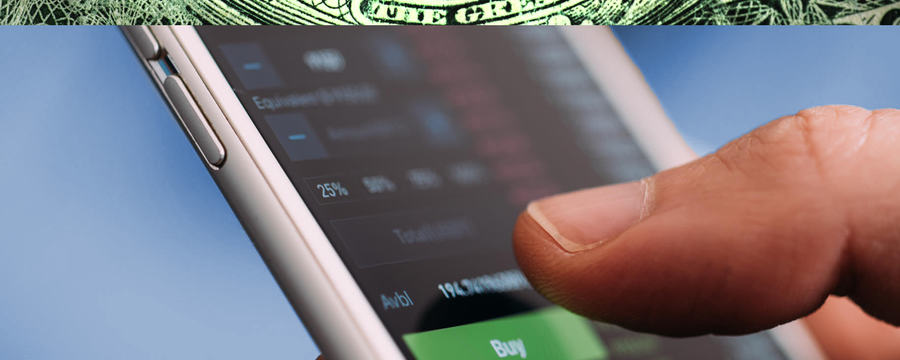
Reach your personal and professional goals
Unlock access to hundreds of expert online courses and degrees from top universities and educators to gain accredited qualifications and professional CV-building certificates.
Join over 18 million learners to launch, switch or build upon your career, all at your own pace, across a wide range of topic areas.