Home / Language / Care / Corpus Linguistics: Method, Analysis, Interpretation / Statistics meets corpus linguistics
This article is from the free online
Corpus Linguistics: Method, Analysis, Interpretation
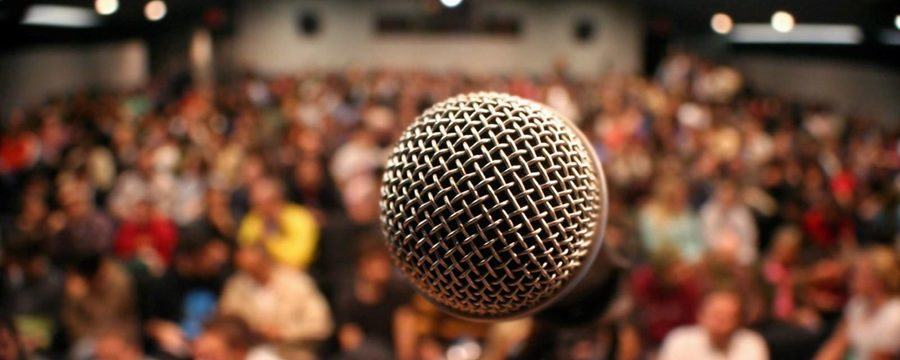
Reach your personal and professional goals
Unlock access to hundreds of expert online courses and degrees from top universities and educators to gain accredited qualifications and professional CV-building certificates.
Join over 18 million learners to launch, switch or build upon your career, all at your own pace, across a wide range of topic areas.