Data hires for the organization
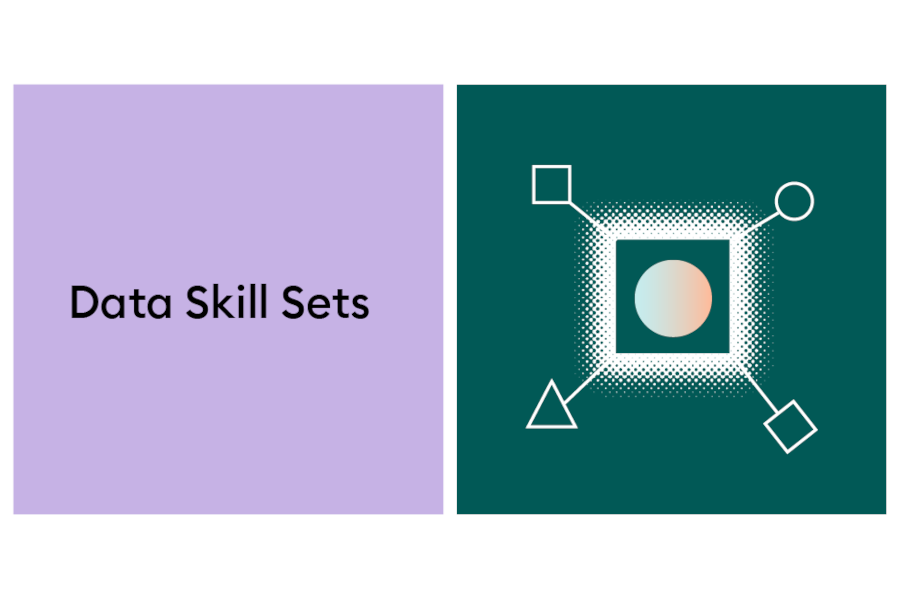
Share this step
To build and use the infrastructure we’ve just discussed, you need people. Data analytics is a tool, and it requires humans to set up these tools, use them, interpret their findings and make decisions on what to do with this information.
And every business focused role needs to acquire data analytics skills, not just our data teams. Just as all business-facing employees had to learn how to use PCs, emails and word processing applications when those became mainstream in the workplace, we now all need to develop our data analytics skills.
However, just as we didn’t have to learn how to build a PC or program the applications we needed, we won’t need to be able to build or maintain the necessary systems and processes because there are people who’ve trained for years to acquire these specialist skills.
Organizations will need to recruit some of these roles, so it’s important for us to understand what they are and where they’re needed in our data analytics journey. As you progress through the data analytics stages and start adding more complexity, the roles and skills an organization requires grow and change.
We’re going to discuss:
- Data engineering roles
- Data engineer
- Data architect
- Data administrator
- Data analysis roles
- Data analytics manager (or data analytics consultant)
- Data analyst
- Data science roles
- Data scientists
- AI and ML engineers
- AI and ML scientists
- Chief Data Officer
We’ll start with the data engineering roles.
Data engineers, architects and administrators
The foundations for any data analytics infrastructure rest on the people and the technology they build and maintain that allows you to capture, collect and gather data. These same people also build the infrastructure that ensures reliable data flows, pipelines, and accessible and connected data storage.
Because of this, one of the first data specific hires would be a data engineer, or a data engineering team. These roles enable data access and value capture. In other words, their ultimate goal is to make data accessible so that organizations can use it to evaluate and optimize their performance. They straddle descriptive and diagnostic analytics and then all the way through predictive and prescriptive analytics.
If a data engineer is working at a smaller company, they’d likely be a generalist taking on a greater variety of data-related tasks, including assisting with the data visualization and reporting requirements. At a large organization, or one with a more mature data analytics set up, a data engineer is more likely to specialize in building data pipelines and maintaining databases.
Whether it’s only a single person, where all positions are rolled into one, or a larger team, the field of data engineering includes the following positions:
- Data architect: Data architects have the capability to “put order into data chaos.” Data engineers assist the data architects to build the working framework for data search and retrieval.
- Database administrator: A database administrator focuses on creating and improving queries, optimizing the database to run as efficiently as possible, maintaining backups, and ensuring data integrity.
- Data engineer: A data engineer builds and maintains the systems that allow you to capture, collect and gather data.
Data engineering teams:
- Build and maintain the systems that allow us to access and interpret data
- Work closely with the data teams to understand the business and data requirements
- Turn these requirements into a delivered solution that supports business information systems and applications
- Build systems that collect, manage, and convert raw data into usable information for data scientists and business focused teams to interpret
- Are responsible for maintaining the data analytics infrastructure
- Validate and update data integrity and quality
Now we’ve looked at what skills are covered by the data engineer or data engineering team, let’s move on to what a data analytics manager does. Data engineers and data analytics managers are both required to understand the business and data requirements of the organization. However, while a data engineer works closely with the data teams, a data analytics manager works with all the teams throughout the organization.
Data analytics manager
A data analytics manager takes the business requirements and translates them into actionable instructions and directions for the data analytics teams. This is an important role as a data strategy must be led by the organization’s business objectives and requirements.
Although the data analytics manager works across all the stages of data analytics, their role becomes more important as the complexity of the data analytics process increases. For example, the process is fairly straightforward at the descriptive analytics stage as we’re simply cataloging “what happened”.
As we start to ask “why did it happen?” at the diagnostic stage, the data analytics manager works with the business teams to decide which variables to look at in order to draw the necessary correlations to answer this question.
Some organizations may decide to bring in a data analytics consultant to perform this role initially if they aren’t able to resource this as a full-time position.
In small- to medium-sized businesses, the data analytics manager may be the most senior data role. This is usually the case if the business is currently only using either descriptive analytics or diagnostic analytics, and business intelligence.
In larger organizations or ones that have a more mature data strategy using predictive and prescriptive analytics, the data analytics manager still has an active role working with the business teams, but they would also be working with data scientists, under the chief data officer.
In summary, a data analytics manager:
- Is responsible for working with the business teams to decipher which data sources are needed
- Works with the data engineers and data architects to ensure the right data is being captured and processed correctly
- Works with both the business-focused team members and data analytics specialist skills in order to ensure data analytics projects are run correctly and are focused on the organization’s goals and purposes.
Data analysts
When the data flows and infrastructure are in place, the next stage is to enable us to actually use the data, which is where the data analysts come in. We’ve briefly touched on what they do, now let’s get more detailed. Once the data engineers and architects have prepared the systems and set up the data analytics infrastructure, the data analysts are able to “translate” the data, by helping organizations to gather meaning from the information, i.e. the data they’ve gathered.
It’s at the insight stage (diagnostic) that data analysts’ contributions and value start to really show as they help make sense of the data. However, the data analysts work with the data all the way through the process, from descriptive to prescriptive analytics. So a data analytics project that starts at the prescriptive stage would still require data analysts to prepare the data.
In summary, the data analysts:
- Organize and transform data into usable and understandable structures
- Clean and prepare data for analysis
- Interpret data-sets, looking for trends and patterns
- Create data analytics reports for the organization’s business teams
- Identify opportunities for improvements in the current data capabilities
- Develop policies for data governance
Descriptive and diagnostic analytics are useful to organizations as they help make sense of the past in order to bring operational efficiencies, as well as a more connected and holistic view of the organization. As we start to move toward looking to the future we add a new level of complexity. To deal with that complexity organizations will need to hire data scientists.
Data scientists
Although some organizations may bring in data scientist skills earlier than the predictive and prescriptive stage, smaller organizations may not require this level of expertise before they’re ready to make the investment to advance to predictive and prescriptive analytics.
The data science team is made up of:
- Data scientists
- AI and ML engineers
- AI and ML scientists
These are highly specialized roles. The data scientist team allows the organization to answer the questions: “What is likely to happen next?” and “What are some of the things we can do as a result?”
In summary, the data science team:
- Extracts insights from large data-sets in order to solve complex problems
- Spends time understanding the industry and business requirements
- Uses computer science, statistics, ML and AI to optimize and learn from a wide range of data sources
- Predicts what might happen next, and then forecasts some of the things that the organization can do as a result
As the focus shifts toward future planning, the ability to work through multiple permutations requires increasingly more complex and powerful technology, algorithms, and AI science. This is both resource heavy and expensive, so it’s important to weigh the costs and resourcing implications against the projected benefit to the organization.
The next role we’re going to talk about is the chief data officer. Like the data analytics manager, the chief data officer has a very good working knowledge of data analytics and works with cross functional teams to direct the data strategy.
Chief data officer
Most small- to medium-sized businesses would not have a chief data officer, unless they have a very mature data strategy that truly leads their business strategy and provides a competitive advantage. Larger organizations that rely on greater quantities of data will increasingly require a chief data officer to manage the complexities of being a data-led organization.
In summary, a chief data officer:
- Is a C-suite role
- Creates business value from data analytics
- Is responsible for implementing the data strategy
- Makes decisions regarding the cost-benefit analysis of the resourcing decisions of the data function with the rest of the leadership team. For example, they’ll make decisions regarding whether to buy “off the shelf” solutions versus building solutions in-house
- Acts as a bridge between the business function and the specialist data analytics skills
- Focuses on both operational and strategic decision-making
That’s an overview of some of the specialist data skill sets that an organization is going to need if they’re to become data-led. Let’s see where these skill sets fit within the diagram we’ve seen previously.
Technical skills
It’s easier to pinpoint at what stage these technical skill sets need to be resourced because they have specific skills that relate to each of the different types of analytics (descriptive to prescriptive).
- Data engineers: As they set up the data analytics infrastructure, the first specialist data skill sets an organization will need are the data engineers
- Data analysts: As organizations start to use the data analytics structure to demand more from their data, data analysts will be required to manage the increased level of complexity
- Data scientists: When organizations start to look into the future, with predictive and prescriptive analytics, they’ll need to bring data science roles into the organization
Technical and business skills
Certain roles act as both a bridge between the data team and business team so they would be brought in at different stages depending on the organizational resources and requirements. Because these roles vary from organization to organization, they don’t fit clearly into the diagram above. These roles include:
- Data analytics manager: The data analytics manager is usually brought in as the team grows and the complexity of the data analytics process increases. They will be the main point of contact between the business and data teams until a chief data officer is brought onboard
- Chief data officer: It is most likely that organizations would onboard a chief data officer to lead the data strategy when the organization is using predictive and prescriptive analytics, and is truly data-led
Which roles are required for each organization will depend on where they are within the data analytics ecosystem. We covered what the data analytics ecosystem earlier, but how do we know where we currently sit within that ecosystem? Knowing that will help us identify our starting point for moving forward in our data analytics journey.
But before we look at where to begin, let’s take a moment to review what we’ve covered so far with a quiz.
Share this
Introduction to Business Intelligence and Data Analytics
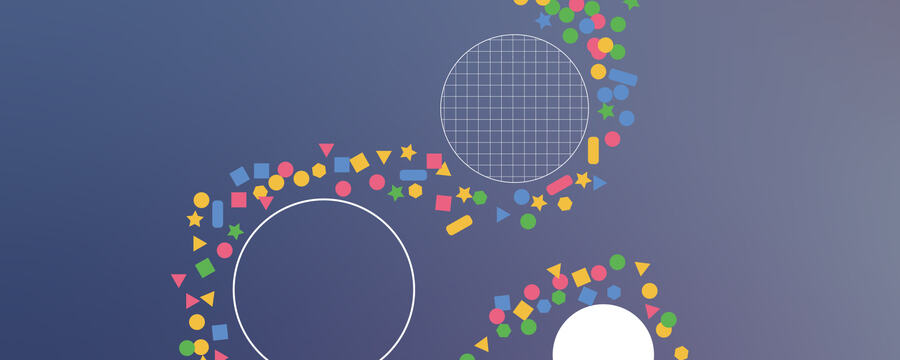
Introduction to Business Intelligence and Data Analytics
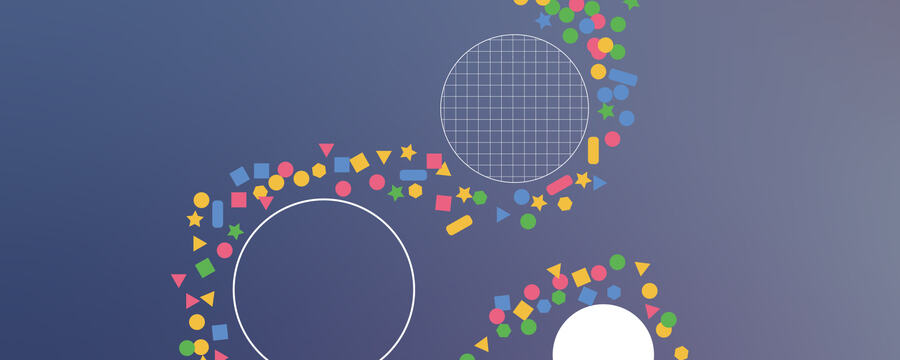
Reach your personal and professional goals
Unlock access to hundreds of expert online courses and degrees from top universities and educators to gain accredited qualifications and professional CV-building certificates.
Join over 18 million learners to launch, switch or build upon your career, all at your own pace, across a wide range of topic areas.
Register to receive updates
-
Create an account to receive our newsletter, course recommendations and promotions.
Register for free